AI in Fintech and the Future of Financial Services
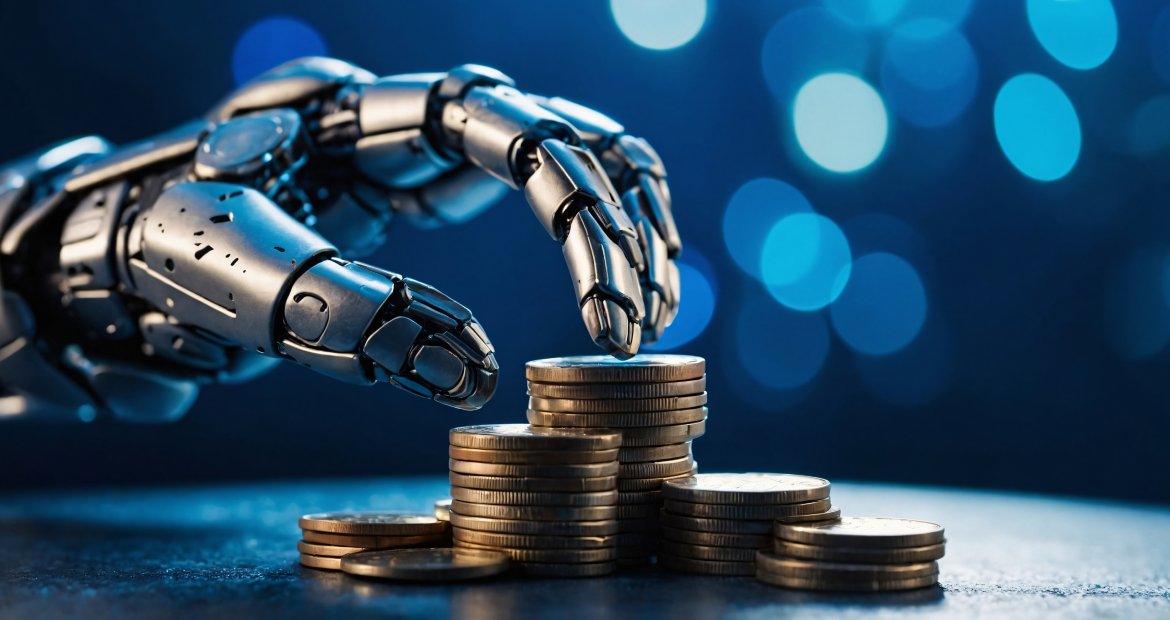
AI glossary: Machine learning, deep learning, natural language processing
Before we go into too much detail, let’s take a minute to talk about AI-related terms that are often used interchangeably but have particular meanings. People tend to call lots of things artificial intelligence, but in fact AI is an umbrella term that embodies many subsets of technologies including machine learning (ML), deep learning (DL), and natural language processing (NLP).
Though each subset has a specific focus, these technologies aren’t mutually exclusive – quite the opposite, they’re interconnected and often combined. To get a better understanding of the relationships between different AI technologies, have a look at the diagram below:
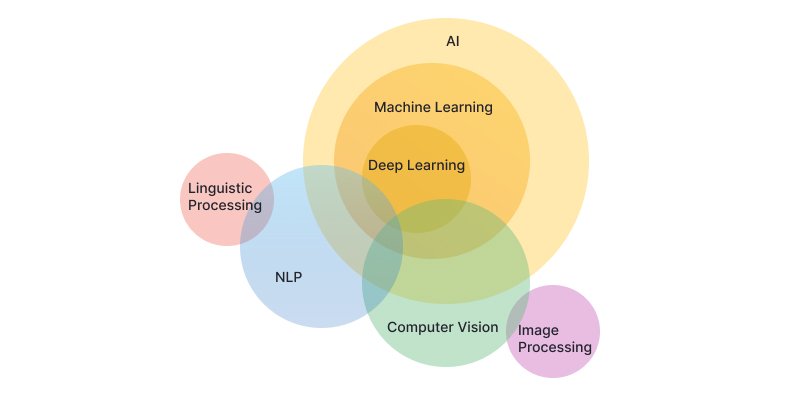
Machine learning
According to Precedence Research, machine learning is expected to become a 770-billion-dollar industry by 2032. But it’s already very much here today, so let’s get into it.
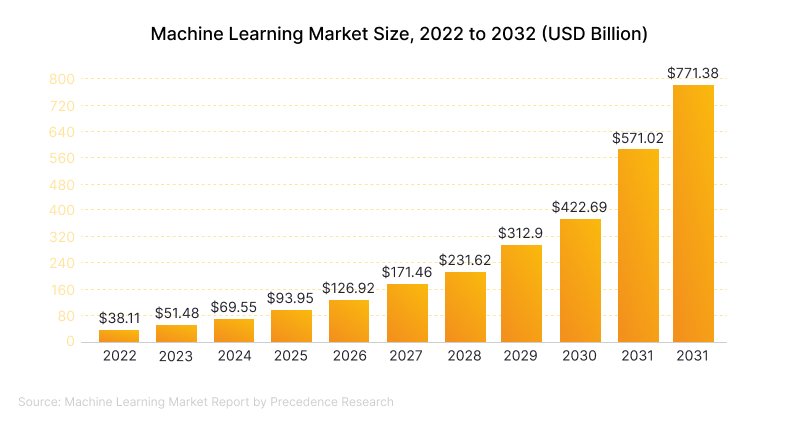
Source: Machine Learning Market Report by Precedence Research
Machine learning is the largest subset of AI, focused on self-learning algorithms that derive knowledge from datasets to predict outcomes. The term machine learning was coined by Arthur Samuel, an engineer at IBM, back in 1959. Before that, artificial intelligence was a study of mechanized or formal reasoning. The idea behind it was applying logical rules and structures to solve mathematical problems. Samuel was the first to define machine learning and take the AI concept a step further. He designed the first program that could play checkers. It analyzed the board by looking as many moves ahead as it could, then made the move with the best projected outcome. It also learned from experience – the program’s algorithms transformed raw data into actionable knowledge to make predictions. So the more the program played, the more it learned – the same as human minds learn, by trial and error. IBM specialists took Samuel’s work even further and eventually created a chess program that could compete with human chess players. Actually, it did. It even beat the world’s chess champion, Garry Kasparov, in 1997.
Machine learning is very much part of our everyday life. Just have a look at some real-life examples of ML and see for yourself:
- Image recognition. Machine learning can recognize and interpret the visual world. For example, Instagram and Facebook use ML algorithms to recognize a user’s friends and automatically recommend tagging them in photos.
- Recommendations. Did you ever wonder how Spotify or YouTube Music know your musical taste so well? Or how eBay provides recommendations tailored to your interests? Or how Indeed can match job seekers with job opportunities? The secret is a recommendation system built on machine learning.
- Financial transactions. With millions of credit card transactions processed every day, it might seem difficult to monitor for fraudulent payments. But not for fraud detection algorithms. They have great potential to recognize suspicious online transactions and flag them for further investigation.
- Cybersecurity. Machine learning is not only great at identifying questionable purchases or payments; it can also detect and respond to cyber attacks and intrusions.
- Healthcare. Around one in ten vascular events, infections, and cancers are misdiagnosed. With machine learning, diagnostic errors can be significantly reduced. For example, today, ML systems are trained to recognize tumors that are hard to see with the human eye. This can not only improve accuracy in radiology imaging and reporting but also provide radiologists with time to focus on suspicious cases. Other use cases of ML in healthcare are early lung cancer and bone fracture detection.
Deep learning
Another subset of AI is deep learning (DL). To be more precise, DL is a subtype of ML that focuses on neural networks, which consist of dozens of layers of interconnected nodes (or neurons) — similar to the human brain. Each layer processes inputs from the previous layer, then passes outputs to the next layer, thus extracting higher-level features and patterns piece by piece.
Deep learning can be used in a whole range of apps for purposes such as:
- Image and audio recognition
- Translation
- Natural language processing
- Analytics and forecasting
- Recommendations
- Self-driving vehicles
Natural language processing
Natural language processing, or NLP, is a subtype of deep learning. NLP allows a system to understand human language (both spoken and written). It works with unstructured text, such as add bread and butter to my shopping list. Humans understand what this means, but for a computer, this piece of text is considered unstructured. Structuring it means representing this same information in a way that the software can process it. In this example, structuring the text would mean creating a shopping list as an element and creating sub-elements for bread and butter.
The job of NLP is to translate unstructured information into structured information, and vice versa:
- When you go from unstructured to structured information, that’s called natural language understanding (NLU).
- When you go from structured to unstructured information, that’s called natural language generation (NLG).
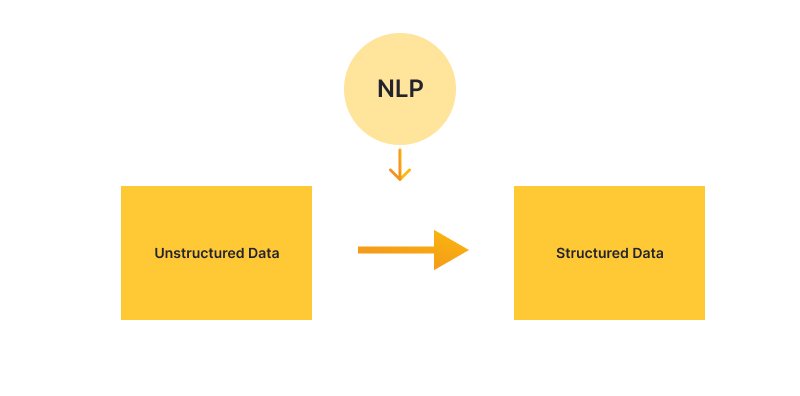
Today, NLP is used in all sorts of AI apps, including for:
- Machine translation. When we translate something from one language into another, it’s important to preserve the original meaning and intent of the text. Word-for-word translations often don’t work, as we need to understand the overall structure and context of what’s being said. NLP is very helpful here.
- Virtual assistants and chatbots. Great examples of virtual assistants are Siri, Alexa, Google Assistant, and Cortana. These voice services can interpret human speech, execute commands, and respond through synthesized voices. Chatbots are similar but use written language.
- Sentiment analysis. This is when a system takes text and determines the sentiment it expresses. A great example of where sentiment analysis might be applied is to understand the sentiment within a product review. Is the review positive, negative, or neutral? Is it sarcastic or serious? NLP can tell you.
- Spam detection. NLP can analyze emails by scanning text for known spam indicators such as poor grammar, overused words, or inappropriate claims of urgency.
AI in fintech by the numbers
When taking on a serious project, it’s important to talk about statistics because real numbers show real interest in the industry and technology. Before we get into real-life AI use cases for fintech software development, let’s have a look at the current state of the financial industry and the impact of AI on it.
- The United States has the largest number of fintech companies. As of January 2024, there were more than 13,000 fintech companies in the US, which is 1,500 more than a year earlier. Additionally, the US reported the highest number of fintech unicorns globally in 2023, with the United Kingdom ranking second. [Source: Statista]
- The largest fintech companies are headquartered in the US and China. They include Visa, Mastercard, Intuit, and Tencent. [Source: Statista]
- Adoption of AI (i.e. embedding at least one AI capability in business processes or products) across the world has increased from 20% in 2017 to 72% in 2024. The majority of those surveyed by McKinsey in 2023 reported they were using AI for two or more functions. [Source: McKinsey]
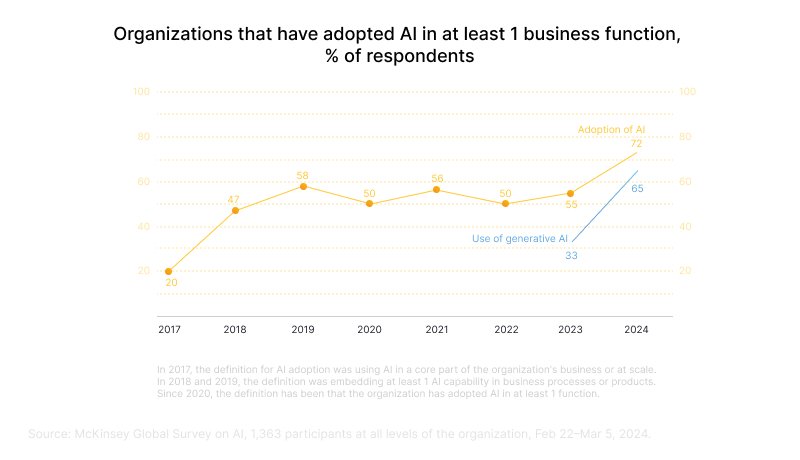
Source: www.mckinsey.com
- The size of the global AI fintech market is estimated to grow at a CAGR of 2.91% from 2024 to 2029, reaching $50 billion. [Source: Statista]
- Among the largest US and European retail banks, Capital One scored the highest on the AI readiness index in 2023. [Source: Statista]
Top use cases of AI in financial services
Finance and banking have embraced artificial intelligence for a long time. In 1989, computer scientist Yann LeCun made important contributions to the AI world in banking, specifically with convolutional neural networks (CNNs), an area of deep learning that specializes in pattern recognition. Yann built the base for lots of AI technologies we now take for granted. One of his early projects was a neural network that helped computers recognize images and even handwritten characters. Did you know that by the end of the 1990s, 10% to 20% of all US checks were already processed by computers? At the same time, financial services institutions started using AI to detect credit card fraud.
Companies in the financial services industry and the retail industry invested more in AI in 2023 than did companies in other industries. in AI-powered technologies. In particular, banks spent $20.6 billion on AI in 2023, and annual AI investment is estimated to surge to $84.99 billion by 2030, growing at a CAGR of 55.55% according to Statista.
No doubt artificial intelligence is transforming financial services. Let’s see how AI is used in the industry today and what its future potential is.
Fraud detection
For businesses, fraud is especially scary — it isn’t only about the company losing money but also about customers losing trust in the company or its services. That’s why detecting fraud and finding effective ways of fighting financial crimes is a critical priority for the fintech industry.
Today, many financial institutions benefit from machine learning for their fraud detection processes. ML can see beyond what human analysts can. It has the potential to make nuanced distinctions between normal and suspicious behavior and thus find red flags.
The most common types of fraud that such systems detect are payment/transaction fraud, new account fraud, account takeovers, promo abuse, identity theft, and phishing.
Detecting fraud with machine learning includes a few steps:
- #1 Understand the baseline. Your model needs to understand what behavior is standard or normal to identify deviations from that baseline and recognize signals of suspicious activity. For this, you need a starting point for analysis (i.e., a solid foundation of historical data). In the financial services context, historical data can be:
- Past transactions (both legitimate and fraudulent)
- Transaction features (purchase amount, exact time of transaction, payment method, credit card number)
- Customer behavior (order history, location data, typical behavior for users in the segment)
- #2 Preprocess data. Still, not all historical information may be important when looking for fraud. Therefore, a data analyst needs to go through each piece of data and mark those with the most predictive power. At this step, a data engineer creates new variables or features to capture relevant data about suspicious activities.
Behavioral analytics comes in handy here. You can divide your customers into groups so the system can identify if one customer’s behavior fits the behavior of the group as a whole. By doing so, you can see if a specific behavior is typical for people in a given segment and detect unusual activity. For example, fraudsters usually make transactions during non-business hours, but (!) late-night shoppers might as well. Once the system considers all features and their predictive power, it generates a fraud score, indicating the probability of fraud. The system calculates how likely it is that a purchase is fraud. When the fraud score is low, the system marks the purchase as suspicious, to be reviewed by the business or customer.
- #3 Set up your model. Here comes the magic. At this step, you train your model to learn patterns and make predictions based on your datasets (preprocessed and feature-engineered data prepared in the previous steps).
What’s important is that you need to revisit and update your fraud detection rules to stay ahead of evolving fraud tactics. For example, even a decade ago your bank would ask you to inform them before traveling or your credit card could easily get blocked when used abroad. Today, transactions made in distant locations don’t always indicate fraud. So you’ll need to train your model to combine different transaction attributes to predict what payments are valid.
- #4 Train and evaluate. In the end, you need to test your fraud detection model in a testing environment that simulates real-life scenarios to see how it performs.
Globaldev’s experience in fraud detection using AI
Recently, we worked on a project called Bounce. The idea behind Bounce was to avoid losing sales to willing customers by analyzing initially declined payments and identifying those that the system could recover. With Bounce, businesses can reduce their churn rate, gain more repeat buyers, and improve their KPIs.
Credit risk management
Traditional credit scoring methods are criticized for being biased. Credit decisions can be influenced by various factors including age, gender, and race. With AI credit scoring, this isn’t a problem anymore. Artificial intelligence can provide fair and objective credit analysis, providing equal opportunities for all borrowers. ML algorithms can be used to analyze vast amounts of data and make accurate lending decisions while reducing bias. Since the algorithms are designed to learn from data and recognize patterns, they gradually and continually refine their models to improve accuracy over time.
Globaldev’s experience in credit scoring with AI
Tarya is a P2P investment platform that is challenging the traditional banking system by offering an innovative solution for secured social loans, bringing together borrowers and lenders without an intermediary.
Tarya uses AI algorithms to distribute investments across dozens of different loans to optimize risk diversification. Additionally, Tarya uses algorithms to recruit borrowers:
- First, the system verifies and analyzes data on a borrower by going through over 300 sources.
- Then, the system evaluates the applicant’s personal characteristics using behavioral science principles.
- Finally, the system looks into the applicant’s historical credit data to forecast repayment ability.
Chatbots and virtual assistants
AI-powered chatbots are revolutionizing how businesses function and financial institutions are no exception. Intelligent chatbots in banking not only manage customer interactions 24/7; they can also help customers open a new account and forward complaints or support requests to the appropriate customer service department.
Predictive analytics
AI is revamping the way financial institutions leverage and analyze data. Artificial intelligence can extract valuable insights from vast amounts of data and see patterns and market trends that were previously invisible. This allows for more thorough and accurate risk assessment, smarter investments, and a better understanding of market dynamics. Additionally, artificial intelligence can learn from past data to predict future outcomes and trends with remarkable accuracy. This can be a great advantage in areas such as credit risk modeling and stock market forecasting.
Benefits of AI and ML in fintech
When you decide to embrace a certain technology, it’s important to outline its advantages and disadvantages. For financial services, artificial intelligence means:
- Enhanced efficiency and productivity. One of the key advantages of AI is its ability to process vast amounts of data at incredible speed. Because of this, it significantly reduces the time required to respond to potential threats.
- Fewer errors and greater accuracy. AI algorithms continuously improve over time, adapting to changing realities. For example, algorithms can adapt to new fraud schemes as they emerge.
- Lower operational costs. Artificial intelligence can automate routine tasks that might normally take hours. It can save time and free up your employees to focus on tasks that require a human touch. Additionally, with AI-driven analytics, you can more efficiently allocate resources, prevent unplanned downtime, and reduce business expenses.
- Automated and personalized customer service. With AI-driven customer insights, you can gain a fuller picture of your customers. You’ll be able to understand their preferences, needs, and motivations as well as get an understanding of current market trends. And with AI-driven tools, you’ll be able to deliver highly personalized and automated customer service 24/7!
- Better security. AI greatly speeds up and improves cybersecurity processes, detects potentially fraudulent activities, and flags questionable transactions.
- Accurate financial forecasts. A lot of financial services companies use AI-powered predictive analytics to get a fresh perspective on growth and profitability. Artificial intelligence can analyze multiple factors including historical sales data, market conditions, and customer behavior.
Unfortunately, adopting artificial intelligence is not without challenges. There are quite a few concerns and hurdles around data privacy, the complexity of training AI models, and the development resources required. But with the right strategies and tools, these challenges can be effectively overcome, leading to more secure financial environments.
Looking for fintech software developers to make your AI idea a reality?
For businesses that lack in-house AI expertise, it’s important to partner with experts who understand the ins and outs of the fintech regulatory landscape, compliance nuances, and modern financial security mechanisms.
At Globaldev, we have broad experience developing fintech apps and have all the resources necessary to create an AI application from start to finish. If you’re interested in building your software with us, don’t hesitate to reach out. And if you have any questions regarding fintech software development with artificial intelligence technologies, we’ll be happy to answer them!